From Pixels to Masterpieces. The Unique Evolution of DALL-E 2, MidJourney, and Stable Diffusion
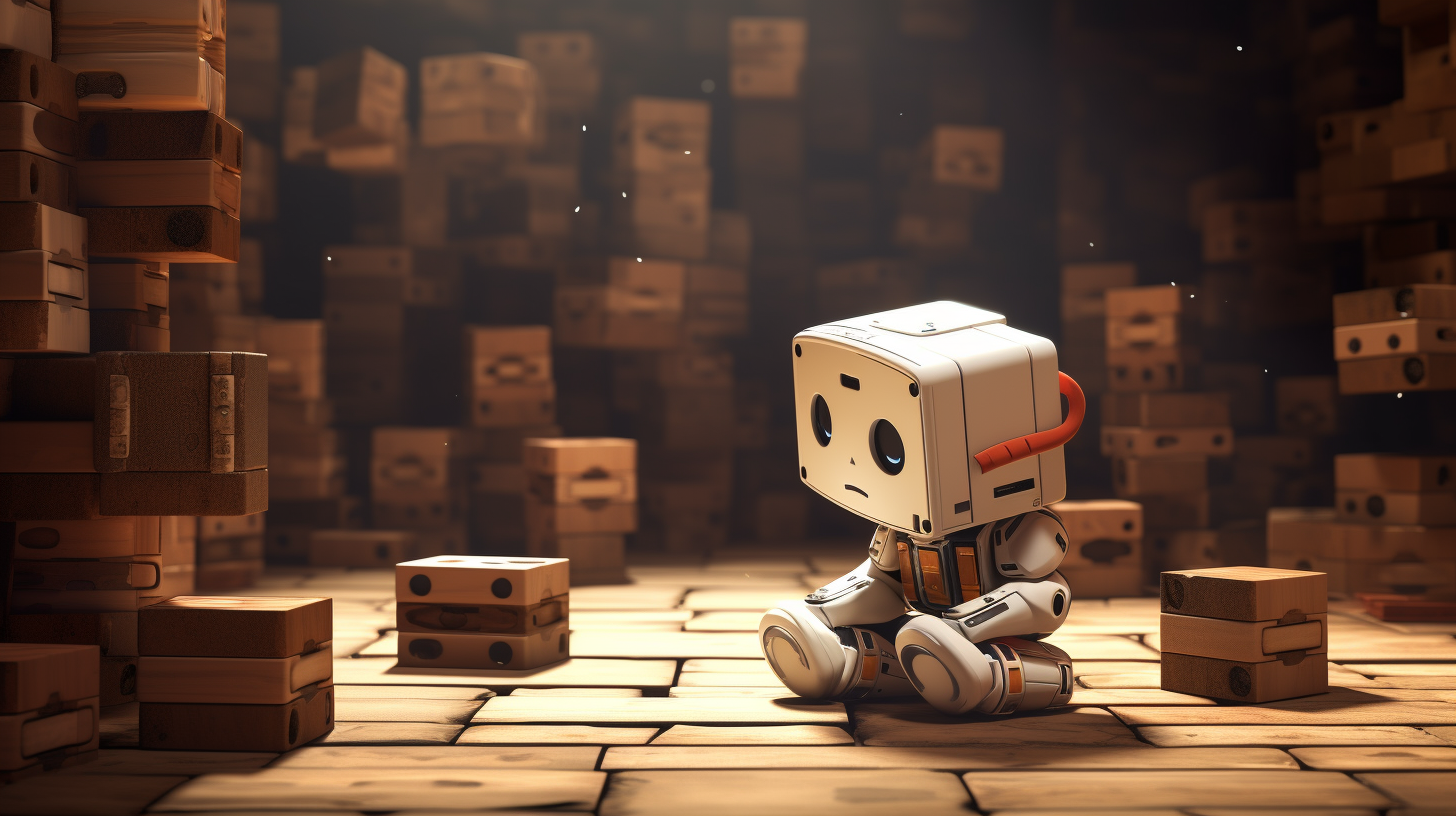
As the first generative AI platform, DALL-E ignited a frenzy among early adopters a year ago. Everyone was seeking the golden ticket to beta-test the groundbreaking technology. The landscape has exploded in the meantime. We are in the middle of a thriving competition among three prominent platforms: MidJourney, Stable Diffusion, and DALL-E, already in its second iteration.
The three were trained on their unique datasets, which gave them unique artistic imprints. They act differently, respond differently, and produce noticeably different imagery. What makes them feel and act like such unique personalities?
The training data
The training dataset defines the core of any AI. The dataset is what provides the AI system with examples of data and their corresponding labels. It creates the learning patterns and relationships, the capacity to generalize and perform, and the potential to create biases in the AI. Whatever the data was trained on gives the AI the foundational knowledge that allows it to learn, perform, and adapt.
MidJourney was trained on image samples "from various sources", to generate brand-new pictures.
Founder David Holz openly admitted that MidJourney did a deep scraping of the Internet, which places the AI under direct fire for copyright issues. The AI specifically leveraged a big web-scraped dataset named LAION developed by a German non-profit.
Stable diffusion was trained on pairs of images and captions taken from LAION-5B
LAION-5B is a publicly available dataset derived from Common Crawl data scraped from the web. It contains the largest dataset among the three, with 5 billion image-text pairs. They’re classified based on language and filtered into separate datasets: resolution, predicted likelihood of watermark and predicted "aesthetic" score (e.g., subjective visual quality). Stable Diffusion used the 2.3 billion English-captioned images subset of LAION-5B as well as another subset of 170 million high-resolution images.
To train DALL-E 2, OpenAI also scraped the Internet for hundreds of millions of captioned images.
The dataset was fed into the model in batches. OpenAI then trained the model to generate images from the text descriptions using supervised learning. During the training process, Dall-E 2 learned to recognize patterns in the data and use them to generate new images. DALL-E 2’s dataset is the smallest of the three. It may have higher quality, but it also has more duplicate and irrelevant images.
While LAION-5B and LAION are publicly available and can be downloaded by anyone, DALL-E 2’s dataset can only be accessed by OpenAI. LAION-5B and LAION are also more diverse than DALL-E 2’s dataset, as they cover more languages, domains, and styles.
Differences in Architecture
There is a striking difference between DALL-E (either iteration), Stable Diffusion, and MidJourney. The three types of architectures, the diffusion model, encoder-decoder, and GAN, shape image-generating AI in distinct ways. The result is a different set of characteristics and capabilities for each model.
Midjourney was trained as a Generative Adversarial Network, or GAN.
This is a type of AI architecture that consists of two interconnected neural networks. One generates random samples. Its objective is to produce realistic data that resembles the images it was trained on. The other acts as a critic who evaluates the generated data. The discriminator provides feedback to the generator, helping it improve its output quality over time. This adversarial dynamic drives the improvement of both networks, resulting in the generator generating more realistic samples while the discriminator becomes better at distinguishing between real and generated data.
GANs offer flexibility in generating novel and creative outputs. They can generate images that possess characteristics from different input sources, enable style transfer between images, and even generate entirely new images based on textual or semantic prompts. GANs provide a powerful tool for generating images beyond simple reproduction.
Stable Diffusion uses a kind of diffusion model, called a latent diffusion model, for its architecture.
A diffusion model is a generative model that starts with a noisy image and gradually refines it until it matches the target distribution.
The diffusion model focuses on the controlled spreading of information and progressive refinement. It’s often used for generating high-quality images with intricate details and textures. The model behind Stable Diffusion allows for fine-grained control over the generation process, enabling the generation of diverse styles and variations.
DALL-E uses an "encoder-decoder" architecture, a similar game between two components of the architecture.
The encoder is like a translator. It takes a picture from the data set and squeezes the defining information from that picture. The encoder will notice the defining details of the picture, like colors, shapes, and patterns, and turn them into code.
The decoder takes the code and uses it to imagine a new picture, putting the shapes, patterns, and colors together based on the code it received. With this picture transformer, you can extract the code from a cat picture, and use it to generate a new cat, with different colors or shapes.
The encoder-decoder architecture is commonly used for tasks like image generation and language generation. It excels at capturing and reproducing the features and structures of the input data and can generate coherent and contextually appropriate outputs. In other words, it shaped the DALL-E versions as the more realistic, down-to-earth AIs.
In Closing
DALL-E, MidJourney, and Stable Diffusion have each been shaped differently as unique image-generative AIs. They have their own strengths and characteristics, distinct architectures, and training methodologies. Their differences pave the way for a rich and diverse landscape of image generation, offering exciting opportunities for creative expression, scientific exploration, and technological innovation. Whether it's DALL-E's encoder-decoder architecture, MidJourney's diffusion model, or Stable Diffusion's GAN-based approach, these AIs are remarkable achievements.