You’ve Noticed. ChatGPT Is Becoming Sluggish, Less Inventive, Less Motivated. What’s Behind the Sudden Change in Attitude?
Users felt a decrease in ChatGPTs performance ever since GPT-4. Experts say we might be looking at a completely different system, splintered into bits so that it saves on energy. AIs are running an extenuating problem with processing power.
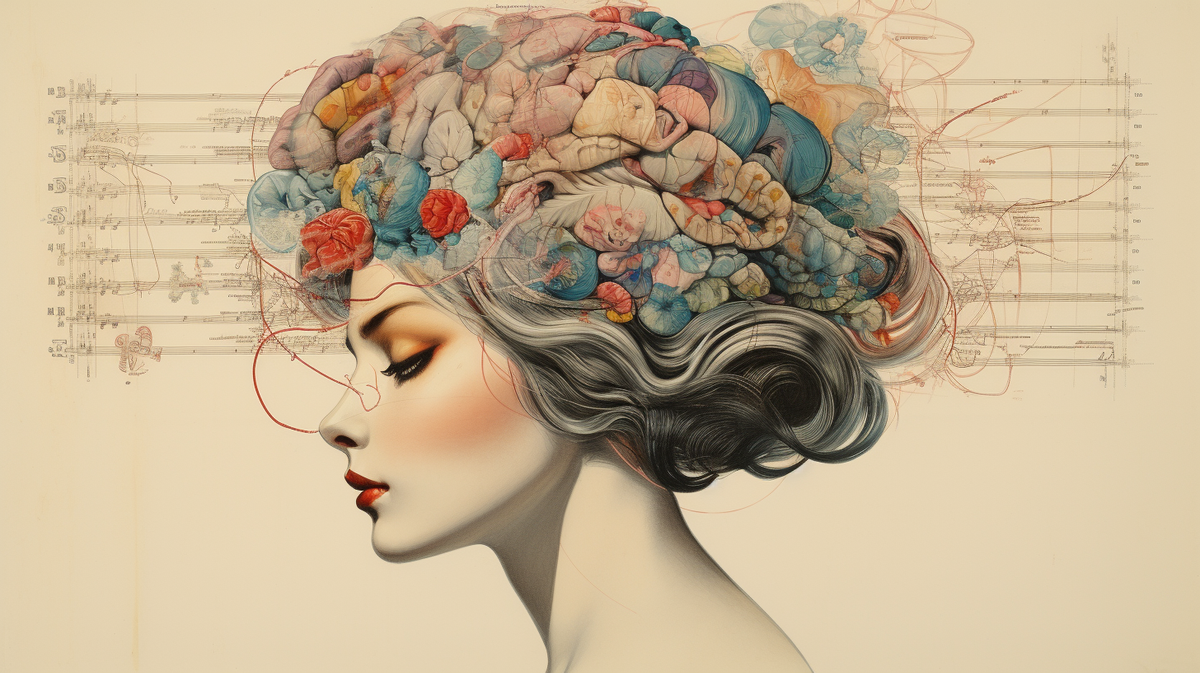
ChatGPT captivated our collective imaginations. This chatbot felt ridiculously good! When the first iteration of a platform looks like it’s going to blow every industry out of the water, what can you expect from the next update?
ChatGPT hasn’t been itself lately
Online users are wondering whether it’s just their imagination, the novelty wearing off, lousy prompting, or an actual platform deterioration.
It’s not just you. ChatGPT has become less agile. There are a few hard-to-quantify issues, like missing the mark on the tone of voice or the general quality of the writing. GPT-4 produces more grammatically-correct noise. Hallucinates more and feels noticeably worse with tasks.
Complains on Reddit have been floating around since March, when the latest update was first released:
“Seems worse with math, GPT 4 incorrectly fixed my list of sig figs” (we found plenty of similar complains about physics, chemistry, programming and history)
“...Yet another answer starts with "buckle up" or it tries to impress me by using something like "bee's knees" to make something sound witty.”
“Its responses have been dull, sometimes limited to just one sentence without any further explanation. This was not the case before.”
“It seems to forget everything after each request or message.”
”It's completely nerfed. Here's some evidence. It can't handle basic facts anymore. Franco German wars 1945-1953??? wtf? It's literally making stuff up.”
“I am finding a looot more "As an AI language model, I am unable to...”
It’s hard to measure the quality of its answers without any data, but GPT-4 is making people talk. The creators have not provided an official explanation for the apparent problem, but several analysts are looking at possible canaries.
Forget About the Black Box Issue and Hallucinations. The Processing Power Is the Tightest Bottleneck for Artificial Intelligence
Running a large language model requires an enormous amount of processing power. Bear with me:
AI models require a lot of data as they are being developed and trained. Models like GPT-3, which has 175 billion parameters, typically require massive computing infrastructure. This includes processors like GPUs or TPUs in parallel, along with high-performance computing clusters. To put it into perspective, an hour worth of training ChatGPT 3 could keep a stadium event running for a few days.
Beyond that, there’s the cost of running the large language model. According to some very rough calculations done in May by Zodhya, running through users' requests for one day costs OpenAI some 260.42 MWh. The energy would keep a mid-size supermarket running for a week. Every single query you make to a language model like ChatGPT costs a thousand times more resources than a Google search.
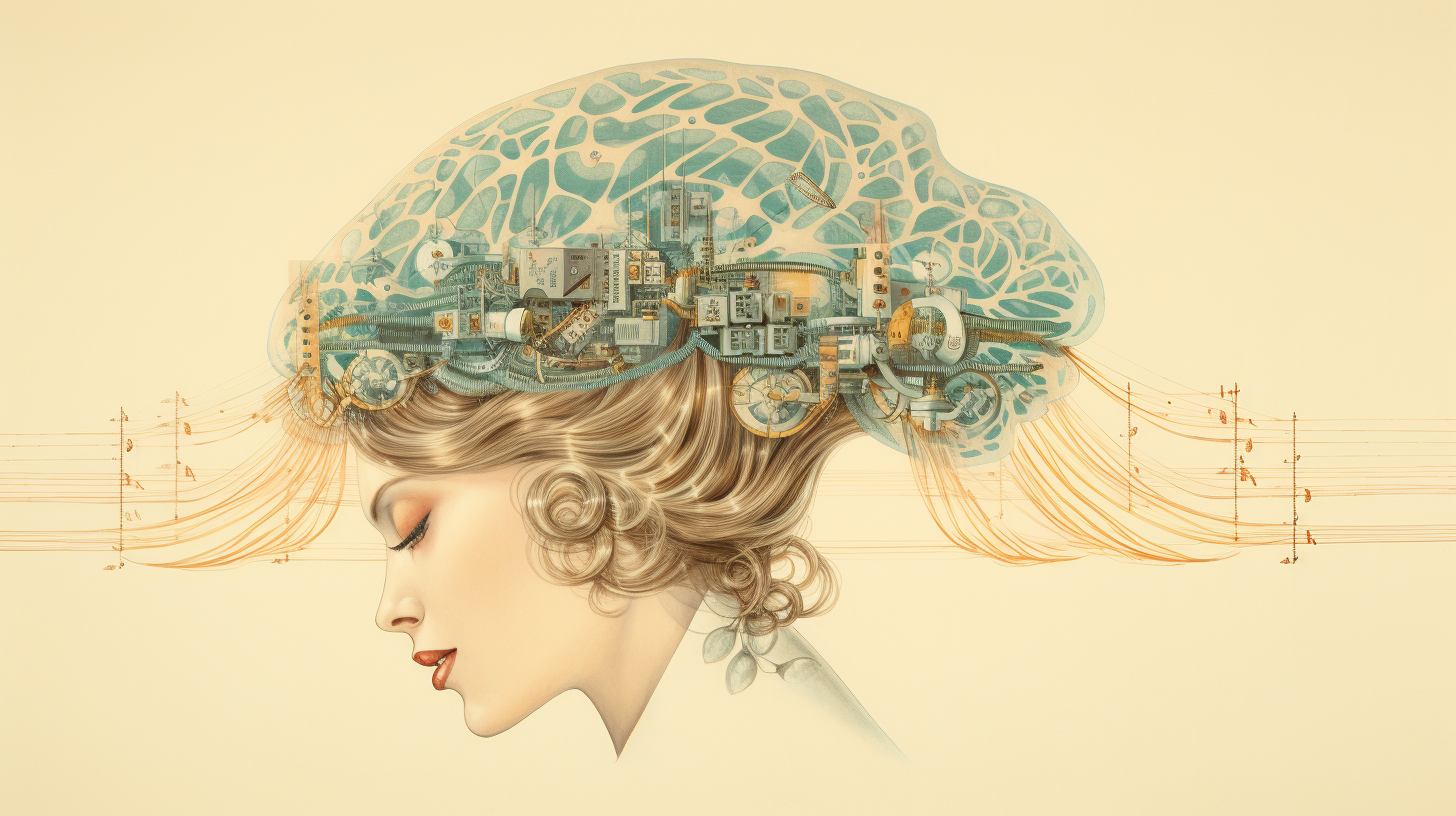
The computational costs of generative AI were highlighted as a national issue in a recent study on artificial intelligence commissioned by the Biden administration.
In the May Senate Subcommittee Hearing, this is what Sam Altman was saying:
“We’re not trying to build up these profiles of our users. We’re not trying to get them to use it more. Actually, we’d love it if they use it less because we don’t have enough GPUs.”
Their model is having a hard time withstanding the amount of power requirements this baby needs. We are dealing with a very hungry wonder-child, in its growing stages. There is an urgent need to build more sustainable systems.
The Next Large Language Model Is Not Going To Be Built by a Student in Their Garage
This immense model requires chips, GPUs, a solid cloud system, and a great deal of processing power. This is not something that you would find in the garage of a nerdy 20-year-old on a summer break. The processing power is comparable to the largest mining complexes of the crypto era, times ten.
There aren’t many companies large enough to support that kind of expense. Google and Microsoft were able to back their language models because of their extensive data centers and dedicated cloud computing. But their models are not yet being efficiently monetized. The companies behind the biggest innovation since mastering fire are losing money on every chat. It’s immensely difficult for OpenAI and Alphabet to keep the models running at full steam. They are not optimized and they are not sustainable.
In front of Congress, Sam Altman said himself that OpenAI and Microsoft are not looking at an ad-based revenue model in the same way Facebook or Google have. Even if they were, there is no amount of advertising that can cover the kind of expense GPT is likely running.
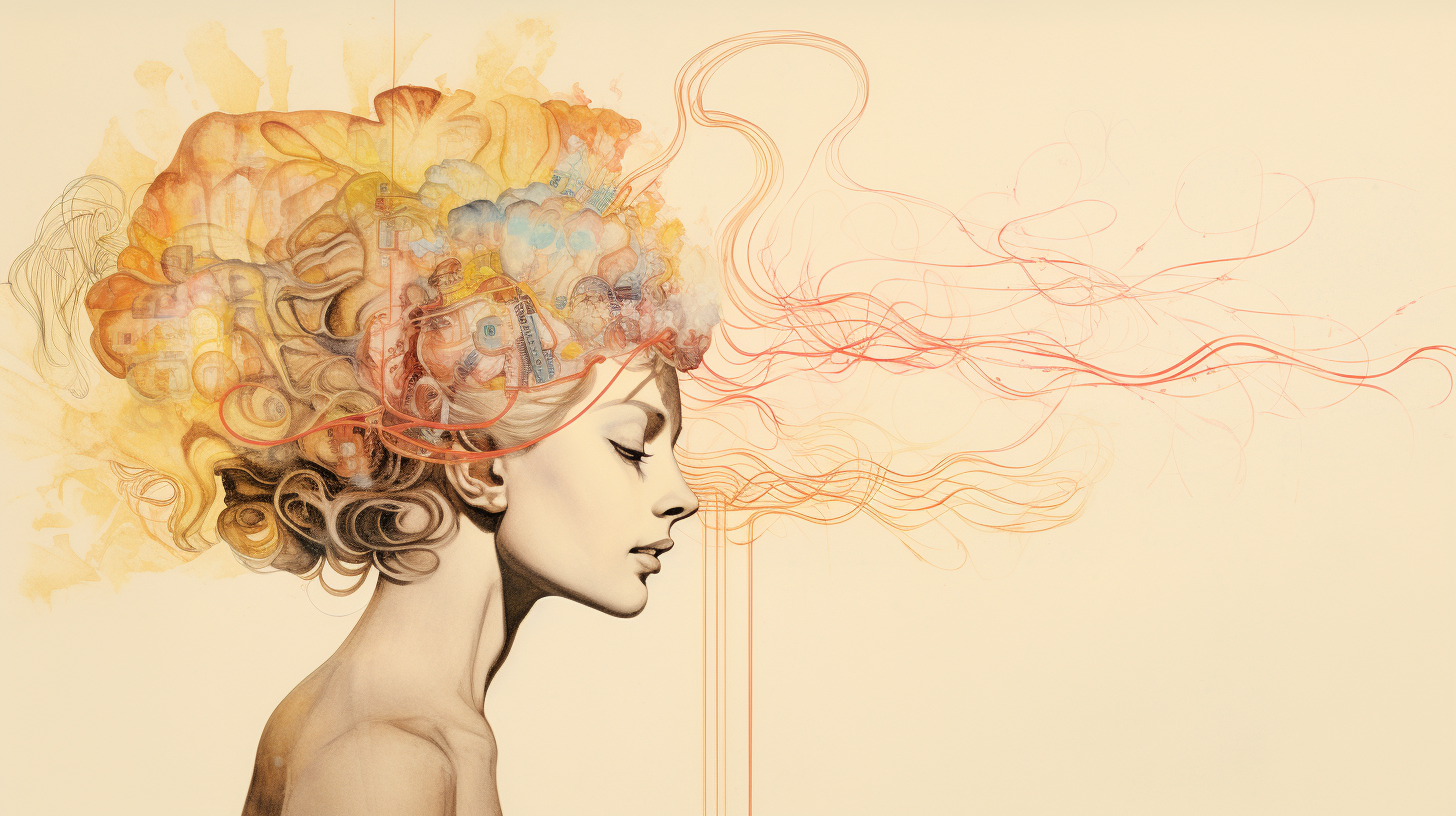
This is the greatest bottleneck in the AI industry. When it comes to this technology, there is a certain quality that emerges only at scale. The more data you feed into the system, the better it gets at generating its output. When that data is limited, the logic, creativity, and responsiveness of the model drops. Dramatically.
In fact, developers have been working on large models since 2012 and only had their current breakthrough recently, once they scaled up their systems. For example, on a small model, a generative AI for images is very, very likely to serve you basic noise, unintelligible shapes, and abstractions. The miracle happened once the system was fed enough data and granted enough processing to assimilate it. It’s like tending to a baby and seeing it grow. It’s dependent on the stimuli it gets.
Turning ChatGPT From a Yacht to a Fleet
The GPT-4 architecture, datasets, and costs have been leaked this month and reveal a completely new architecture, compared to previous models.
Business Insider does a great report on what might be the approach of OpenAI to the processing issue. Apparently, the model has been changed so much that it’s not even the same creature.
Sharon Zhou, CEO of Lamini, a startup that helps developers build custom large language models, analyzed ChatGPT. She says OpenAI changed their approach, by mincing GPT-4 into 16 distinct models that would be less expensive to run. The Mixture of Experts (MOE) approach entails building expert models specialized in their own fields (programming, physics, chemistry, etc).
In an MoE model, a gating network determines the weight of each expert in the output and does the triage. Instead of each query firing up the entire network for answers, it would go through funnels into a single section. This takes less of a toll on their computational power.
"OpenAI is taking GPT-4 and turning it into a fleet of smaller ships," she said. "From my perspective, it's a new model."
Here is a run-down of the leaked information, as reported by Decoder
GPT-4's Scale: GPT-4 has ~1.8 trillion parameters across 120 layers, which is over 10 times larger than GPT-3.
Mixture Of Experts (MoE): OpenAI utilizes 16 experts within their model, each with ~111B parameters for MLP. Two of these experts are routed per forward pass, which contributes to keeping costs manageable.
Dataset: GPT-4 is trained on ~13T tokens, including both text-based and code-based data, with some fine-tuning data from ScaleAI and internally.
Dataset Mixture: The training data included CommonCrawl & RefinedWeb, totaling 13T tokens. Speculation suggests additional sources like Twitter, Reddit, YouTube, and a large collection of textbooks.
Training Cost: The training costs for GPT-4 was around $63 million, taking into account the computational power required and the time of training.
Inference Cost: GPT-4 costs 3 times more than the 175B parameter Davinci, due to the larger clusters required and lower utilization rates.
Inference Architecture: The inference runs on a cluster of 128 GPUs, using 8-way tensor parallelism and 16-way pipeline parallelism.
Vision Multi-Modal: GPT-4 includes a vision encoder for autonomous agents to read web pages and transcribe images and videos. The architecture is similar to Flamingo. This adds more parameters on top and it is fine-tuned with another ~2 trillion tokens.
Is the Resource Issue the Only One That Is Weighing Down the Model?
Most likely this is the number one problem on the Trello board of OpenAI.
This being said, OpenAI has a slate of other concerns on their list, that might spur them into chopping up into the artificial brain. While it might frustrate some users, OpenAI has a very clear stance on keeping the model safe for use. The company is navigating a great deal of responsibility and wielding a powerful disruptor.
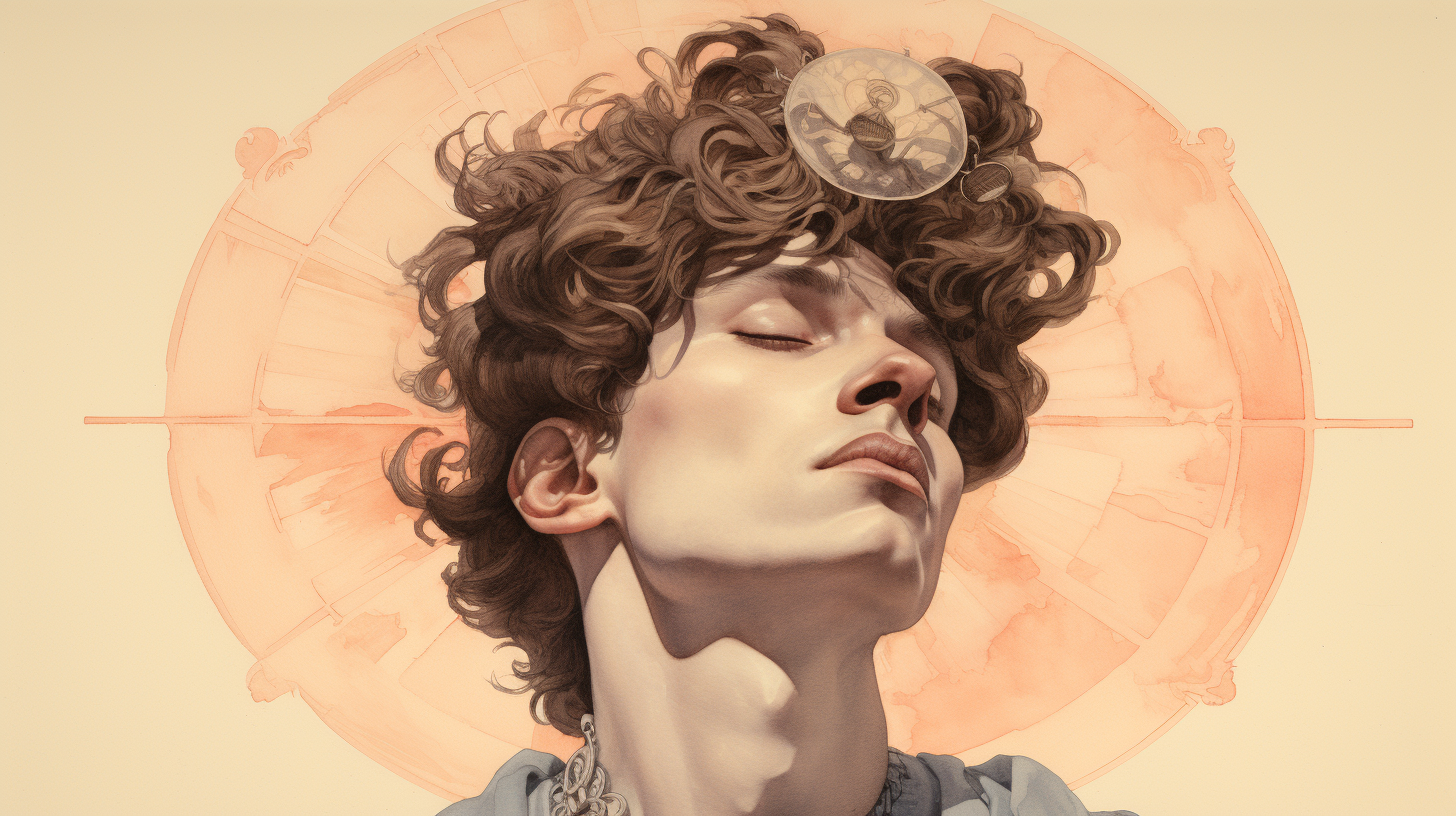
- People have been jailbreaking the language model to bits, making it say spooky, unsettling, or lewd lines. Developers are plugging those holes as they come. One of the scenarios is this makes the model less creative and (I want to put it like this) less trusting around strangers. We went through an in-depth article about Jailbreaks recently and it’s been an absolute joy writing it.
- The company is navigating a legal minefield. It’s very tempting to ask your ChatGPT or your aunt what works best for sore muscles. In many countries (certainly most of the European ones) it’s illegal to say “I’m not a doctor but you should try THIS.” While authorities are not going to come for your hippie aunt, they are certainly keeping an eye on what AI devs are doing.
- The AI's brain works in some mysterious ways (with a strong accent on some, please don’t create a religion around it). There are blind spots where we don’t know precisely what happens. Whenever developers are operating in one area, they run the risk of touching other circuits. This is how you end up with the AI saying “Buckle up!” or staring blankly into space.
While trying to fix certain issues, developers might be running into other snags. Yes, tech has bugs and problems, AIs are not perfect, but hey, my hair isn’t great either and I have the space awareness of a flipped turtle.